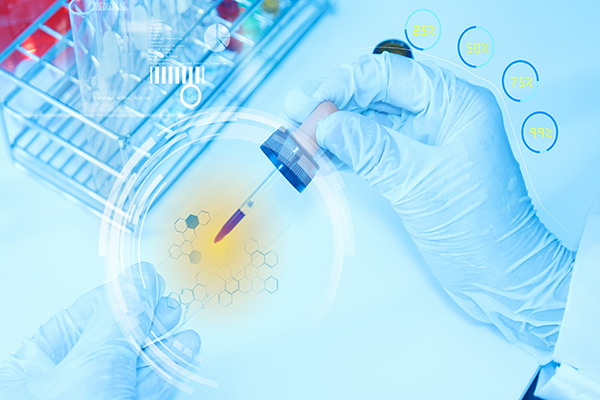
Elizabeth Caro Montero, a researcher at the Universidad Internacional Iberoamericana (International Iberoamerican University, UNIB), is collaborating on a study that proposes a deep learning-based approach for accurate and timely detection of malaria.
Malaria is a dangerous disease that is caused by the bites of infected female mosquitoes. It is infectious not only among humans, but also among animals. It causes mild symptoms such as fever, headache, sweating and vomiting, and muscle discomfort. Severe symptoms include coma, convulsions and kidney failure.
The high burden of malaria cases, especially in Africa, Southeast Asia and the Eastern Mediterranean, has put the effectiveness of manual detection of infected red blood cells to the test. The conventional method of diagnosing the disease involves deterministic examination of blood samples under a microscope. This approach can be challenging due to overlapping cells in thick samples, which can make it difficult to distinguish between healthy and infected cells.
In order to expedite the diagnosis of malaria infections, the use of high-resolution digital cameras or cell phones to capture images of blood samples has been proposed. However, the quality of photographs taken with cell phones can be inferior, making manual interpretation and disease detection using machine learning algorithms difficult.
To overcome these challenges, the use of deep learning algorithms that can rapidly preprocess images and estimate features important for malaria diagnosis and classification has been implemented. Machine learning methods, such as support vector machines, Naive Bayes classifiers, and neural networks, have demonstrated infection detection accuracy between 83.5% and 85%. However, the success of these algorithms is highly dependent on access to well-constructed, discriminative feature vectors extracted by trained professionals.
Therefore, an automated and efficient deep learning-based automated model was developed using red blood cell samples. This approach achieved a high accuracy score of 97.57% for malaria detection. By using fast preprocessing algorithms and automating feature extraction, accurate and reliable diagnosis of the disease can be achieved. The algorithm eliminates the need for manual feature extraction, significantly reducing the workload of pathologists and speeding up the diagnostic process.
This innovative approach combines two essential elements: image analysis and trained algorithms. By training the algorithm with a large dataset of blood sample images, it not only detects and diagnoses malaria accurately, but also assesses the severity of the parasite. This comprehensive analysis benefits both pathologists and patients, providing efficient, cost-effective and reliable results.
This innovative methodology offers a promising solution for regions with a shortage of qualified pathologists. Implementation of this approach has the potential to revolutionize malaria detection, ultimately leading to more efficient management of this global health problem.
To learn more about this study, click here.
To read more research, consult the UNIB repository.
The Universidad Internacional Iberoamericana (UNIB) offers the Master's program in Strategic Management with Specialization in Information Technology. A program that develops the skills and capabilities of students to manage a systems and ICT management position or to lead an organizational change project.