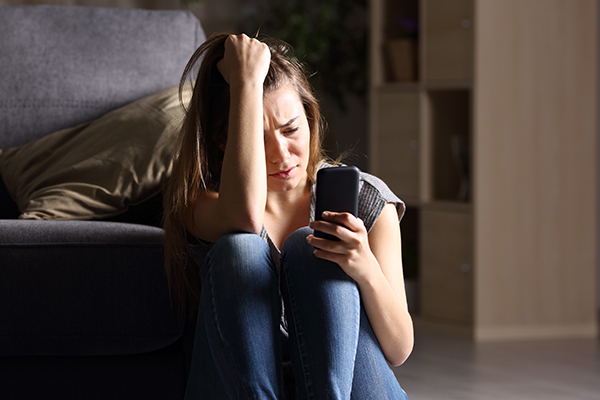
Josep Alemany Iturriaga, researcher at the Universidad Internacional Iberoamericana (International Iberoamerican University, UNIB), is collaborating in a study that develops a new approach to predict the severity of depression in early stages using deep learning algorithms.
Currently, depression is one of the most prevalent mental disorders in the world. It is characterized by feelings of sadness, emptiness and loss of interest in life. It is a disorder that affects people of different ages, genders and socioeconomic levels and can be caused by a combination of genetic, biochemical, environmental and psychological factors.
According to the World Health Organization, approximately 450 million people suffer from depression worldwide, and it is estimated that this figure will increase by 15% by 2030. This condition not only affects a person's quality of life, but also has a negative impact on social and physical relationships. Numerous studies have established links between depression and physical illnesses such as cardiovascular disease, diabetes, accidents, respiratory diseases, cancer and obesity.
In addition, there is a close relationship between alcohol use disorder and depression. Many people turn to alcohol or drugs as a way to obtain relief from symptoms of depression, which can lead to abuse and dependence on these substances. It also increases the risk of suicidal ideation and premature deaths. Depressed people have a twenty times higher risk of suicide than the normal population.
Late treatment of this disorder has a considerable impact on society. Its diagnosis requires several psychological tests performed by experts in psychiatry, as well as interviews, questionnaires and reports from family members and close loved ones. However, it is common for those suffering from depression to delay seeking medical help until the condition has deteriorated.
On the other hand, deep learning has been widely used in fields such as health and medicine, but its adoption in psychology and behavioral sciences has been limited so far. Although there is already research that has used this technology to predict signs of depression, few have focused on severity levels as a multi-class variable.
Algorithms such as Random Forest and Support Vector Machine have been shown to be effective in the early detection of depression. However, one of the challenges is working with unbalanced data sets, where certain classes have significantly more samples than others. This can affect the accuracy of the model.
To address this challenge, in this research, the Feature Group Partitioning (FGP) approach was implemented in the data processing phase, combined with synthetic oversampling techniques such as Synthetic Minority Oversampling Technique (SMOTE) and Adaptive Synthetic Technique (ADASYN) to balance the datasets, in order to improve the accuracy in predicting depression severity.
The results showed that this combination yielded the highest level of balanced accuracy, reaching 92.81%. In addition, the research succeeded in optimizing the training time of the FGP approach for all classifiers used, which represents a significant achievement in this field.
Understanding and accurately predicting the severity of depression is of utmost importance to design personalized treatment plans and provide adequate support to people affected by this mental disorder. This approach and the integration of machine learning algorithms open up new possibilities in the field of depression research.
If you want to learn more about this study, click here.
To read more research, consult the UNIB repository.
The Universidad Internacional Iberoamericana (UNIB) offers the Master's program in Strategic Management with Specialization in Information Technology. A program that prepares students to manage a systems and ICT management position or lead an organizational change project using ICT.