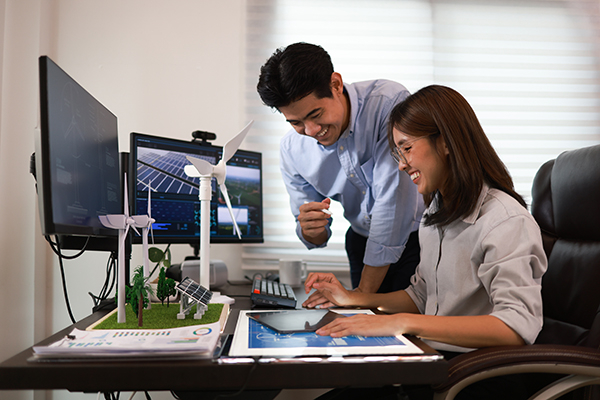
Dr. Yini Miró, researcher at the Universidad Internacional Iberoamericana (UNIB), collaborates in a study that proposes a method based on artificial neural networks (ANN) to predict and estimate energy generation from hybrid wind and solar energy resources in real time.
With the increase in energy demand due to industrial development and contemporary lifestyle, there is a global search for economical and reliable energy resources. In addition, the depletion of traditional energy resources, such as coal and hydropower, and the rising prices of fossil fuels, accelerate the drive towards the use of renewable energy.
This type of energy has gained popularity in recent years due to lower material costs, technological advances, its environmentally friendly nature and favorable government policies. On the other hand, solar and wind energy are accessible everywhere, each with different advantages, and the world is increasingly focusing on utilizing these renewable resources.
Although the most efficient solar panels are still expensive, a hybrid system combining wind turbines and solar panels has been shown to be more effective. However, accurately estimating power generation from these sources can be challenging due to their intermittent nature and variations in intensity between day and night.
To address this challenge, Dr. Miró, along with other researchers, has turned to the power of artificial intelligence. The objective of this study is to contribute to the prediction of renewable resources and to improve the efficiency of hybrid power generation using ANNs. To this end, an innovative method is presented that employs a series of environmental variables that correlate wind speed and solar radiation. These variables include data on temperature, humidity, atmospheric pressure, solar radiation, optimum angle and target values of the velocities.
Through a normalization function and an iterative Levenberg-Marquardt training algorithm, the ANN model was able to reduce the errors inherent in the estimation compared to other currently used methods. The results obtained in the experiments demonstrate the effectiveness of the approach; a prediction accuracy of 2.08% was achieved.
The application of this ANN technique in the prediction and estimation of hybrid wind and solar resources in real time has important practical and economic implications. It enables efficient planning of hybrid system operation, which, in turn, can increase the amount of renewable energy, reduce costs in generation, and increase the sustainability of electricity supply.
In addition to its application in the energy industry, this method also has potential impact in areas such as meteorology, agriculture and urban development planning. The ability to accurately predict wind and solar resources can contribute to the design of policies and strategies that promote the sustainable use of renewable energy sources and drive the transition to a carbon-free economy.
To learn more about this study, click here.
To read more research, consult the UNIB repository.
The Universidad Internacional Iberoamericana (UNIB) offers a Master's Degree in Environmental Management and Audits and a Master's Degree in Strategic Management with a Specialty in Information Technology.